In an era marked by the increasing complexity and scale of IT environments, Artificial Intelligence for IT Operations (AIOps) is a shining example of efficiency, guiding the way to streamline IT processes and operations. To understand when to use AIOps, we’re breaking down what it is and why it matters. We’ll explore some practical AIOps use cases and showcase how leveraging its capabilities can effectively enhance IT operations without triggering unnecessary alerts.
Key takeaways:
- AIOps integrates AI and machine learning into IT operations for enhanced incident response.
- AIOps can be used in several cases, including incident detection, noise reduction, context, continuous improvement, and data integration.
- New Relic Applied Intelligence offers unique features like data integration and transparency in correlation logic.
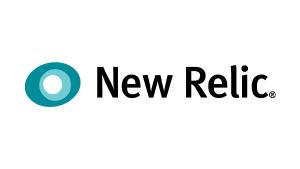
Why is AIOps in demand?
In plain terms, AIOps gives SREs the support they need by proactively detecting anomalies in their environments before they have time to snowball into bigger issues.
Let’s back up a little. Current software development focuses on rapid deployments, butthis constant iteration strains infrastructure reliability. SREs focus on reliability specifically and strive to accelerate incident response to ensure uptime and that service level objectives (SLOs) are met. But they need better ways to monitor and react to issues. With ever-expanding estates, SREs don’t have the time to manually analyze all the telemetry data to find anomalies.
Playing investigator slows their response time and impacts reliability. If they can’t meet SLOs, their entire enterprise is impacted. Enter: AIOps, helping to proactively detect issues, and fast.
AIOps fully supports the philosophy of DevOps because it breaks down data silos. An AIOps platform works best when there are large amounts of data, which can include observational and engagement data, as well as data from third-party tools. A variety of algorithms and machine learning is then applied across the data to find tailored insights that can help teams more quickly identify, diagnose, and resolve issues.
The end goal is to help your teams work faster and more efficiently using the approach that works best. Spoiler: sometimes, it isn’t the most complex machine learning algorithm.
AIOps use cases and examples
How can you use these new tools? What impact will they have on your incident management workflows? There are specific use cases where you can apply AIOps.
1. Incident detection
Adding an AIOps solution expands your toolkit so that you’ll be able to detect problems much sooner. For example, the detection of anomalies can help you keep issues from happening before they start. It’s the most proactive approach to ensure you are aware of the issue before there is any effect on your customers.
2. Noise reduction
Alert fatigue is a huge problem in incident response. A barrage of alerts means you can become numb to all alerts, even if they’re critical. Ideally, you want to suppress low-priority alerts and group alerts that are related. With an AIOps solution, you can correlate, suppress, and prioritize alerts. Thus, your team can focus on issues that are the most critical to reliability.
3. Root cause analysis
Incidents can be complicated, and having a guide to point you in the right direction helps. An AIOps solution automatically analyzes the data for a holistic understanding of the incident. It then provides insight and the context you need to resolve it.
4. Continuous improvement
Past experiences, current usage, and user feedback provide excellent data to help prevent issues that are similar to historic issues, which is crucial for continuous improvement. AIOps trains on this knowledge to continually get smarter and deliver tailored correlations, insights, and recommendations.
5. Data integration
Incident data from any source integrates with your current incident management tools and workflows. The more data you have coming in, the better trained your machine learning will be and the more tailored and useful your results will be. An AIOps solution ingests data, enriches it with context, and sends notifications to the relevant teams or responders in the incident management tools teams are already using. This way, teams don’t waste critical time switching between tools.
6. Cost optimization
By automating and streamlining incident management processes, AIOps helps reduce operational costs. It eliminates time-consuming human error and reduces the need for multiple tools and resources. This not only saves money but also allows teams to focus on more strategic tasks that drive innovation and business growth.
7. Improved customer experience
One of the key benefits of AIOps is its ability to identify and resolve issues before they impact your customers’ experience. By analyzing large volumes of data in real-time, AIOps can quickly detect anomalies or potential incidents and take proactive measures to prevent them from escalating into major problems. This leads to improved service levels, increased customer satisfaction, and ultimately, better business outcomes.
8. Scalability and flexibility
AIOps is highly scalable and can adapt to the ever-changing needs of your business. As your infrastructure grows, AIOps can handle the increased volume of data without compromising performance. It also offers flexibility in terms of deployment options - whether on-premise or in the cloud - allowing organizations to choose the approach that best suits them.
9. Predictive analytics
With AIOps, you don't have to wait for issues to occur before taking action. By leveraging machine learning algorithms, AIOps can predict potential problems and suggest proactive measures to avoid them altogether. This not only reduces downtime but also improves overall system reliability and stability.
10. Enhance the resilience of the entire system.
In today's fast-paced and highly connected world, downtime is not an option. AIOps helps strengthen the resilience of your systems by providing real-time insights into the health and performance of your entire infrastructure. By continuously monitoring and analyzing data from all components - servers, networks, applications, databases, etc. - AIOps can quickly detect any issues and provide recommendations for remediation.
Industry-specific AIOps use cases
AIOPs has gained traction in various industries due to its ability to streamline and improve operational processes. Let's take a look at some examples of how AIOps is being applied in different sectors:
- Banking and finance: AIOps is used for fraud detection and prevention. By analyzing large amounts of data in real-time, AIOps can identify and flag suspicious transactions, providing a more accurate and efficient way to combat fraudulent activities.
- Retail: AIOps is transforming the retail industry by optimizing supply chain management. By utilizing machine learning algorithms, retailers can predict demand and inventory levels, reducing waste and ensuring products are readily available for customers. AIOps can also be used for personalized marketing strategies by analyzing customer data and behavior.
- Healthcare: AIOps is being utilized to improve patient care and reduce costs. By reviewing patient data, doctors can make more accurate diagnoses and provide personalized treatment plans. AIOps can also help with hospital operations by predicting equipment maintenance needs and optimizing staff schedules.
- Manufacturing: AIOps is being used for quality control and predictive maintenance in the manufacturing sector. By examining data from sensors on machines, AIOps can detect any anomalies or potential breakdowns before they occur, preventing costly downtime. It can also help with inventory management by predicting demand and optimizing production levels.
- Transportation: AIOps enables predictive maintenance for vehicles and optimizing routes for maximum efficiency. By analyzing data from sensors on vehicles, AIOps can detect potential issues before they become major problems, reducing maintenance costs and improving safety. It can also optimize route planning by considering real-time traffic and weather conditions.
Why New Relic Applied Intelligence is unique
New Relic Applied Intelligence uses raw monitoring data to power machine learning and can ingest, correlate, and alert on sources outside of New Relic, such as PagerDuty, and more. In this way, users remove silos and get a better big picture of the issue that is occurring with important context around it. And integration with existing incident management tools means that New Relic Applied Intelligence meets teams where they’re already working.
This provides users with:
- Fastest time-to-noise-reduction: New Relic usesautomatic, out-of-the-box correlations that continually self-improve. The platform automatically reduces noise by enriching incidents with context in real-time, includes AI/ML-powered suggested correlations, and frees you from steep learning curves, lengthy implementation and training times, or complex integrations found with other AIOps tools.
- Fits existing incident management workflows: New Relic, the sole AIOps solution meeting you where you are, integrates seamlessly with your current tools. Easily connect them as data sources via New Relic's interface, and it handles the rest by ingesting, enriching, and providing insights on incidents to PagerDuty, Slack, Jira, ServiceNow, VictorOps, and your tools of choice.
- Faster time-to-value: Analyze and act on more data types. While tools like PagerDuty provide escalations based on alerts, New Relic lets you ingest, analyze, and act on multiple data types, like alerts, logs, metrics, and deployment events. You gain better incident context to diagnose and prioritize issues swiftly with New Relic.
- Transparency: Knowing why incidents are correlated builds trust. AIOps tools shouldn’t be a black box. New Relic Applied Intelligence provides full transparency into why and how correlations are performed and lets you tune the system with your own correlation logic (by simply telling New Relic what data to compare and how you want to correlate the data), so you have confidence in the ML models that reduce noise while ensuring that critical signals don’t go unnoticed.
Explore New Relic Applied Intelligence
These AIOps use cases demonstrate how your organization can benefit from adopting an AIOps solution. With proactive anomaly detection, alert noise reduction, issue enrichment, and smarter notification, New Relic Applied Intelligence gets you closer to the root cause and enables you to detect, respond to, and resolve issues faster than ever before.
If you’re ready to learn more about preparing for AIOps, don’t miss our ebook, How to Prepare for AIOps: Four steps for a successful deployment.
Die in diesem Blog geäußerten Ansichten sind die des Autors und spiegeln nicht unbedingt die Ansichten von New Relic wider. Alle vom Autor angebotenen Lösungen sind umgebungsspezifisch und nicht Teil der kommerziellen Lösungen oder des Supports von New Relic. Bitte besuchen Sie uns exklusiv im Explorers Hub (discuss.newrelic.com) für Fragen und Unterstützung zu diesem Blogbeitrag. Dieser Blog kann Links zu Inhalten auf Websites Dritter enthalten. Durch die Bereitstellung solcher Links übernimmt, garantiert, genehmigt oder billigt New Relic die auf diesen Websites verfügbaren Informationen, Ansichten oder Produkte nicht.