Today we’re announcing the integration of Azure Machine Learning with New Relic to help you monitor your machine learning (ML) workspaces, endpoints, and deployment-related metrics, and the availability, performance, and resource utilization of your ML resources. The integration gives you pre-built visualizations and allows you to troubleshoot ML-related issues alongside other infrastructure and application telemetry in one unified dashboard.
The new integration allows you to:
- Keep track of ML workspaces by collecting data on all workspace activities, including quota details.
- Monitor the performance of ML endpoints by gathering metrics on their operational efficiency.
- Get oversight of ML endpoint deployments.
- Get visibility of important performance metrics using a pre-built dashboard that includes job executions, model metrics, quota metrics, online endpoints, and endpoint deployments, as well as CPU and GPU usage per workspace.
- Customize alerts to suit your specific needs.

Visibility into job executions
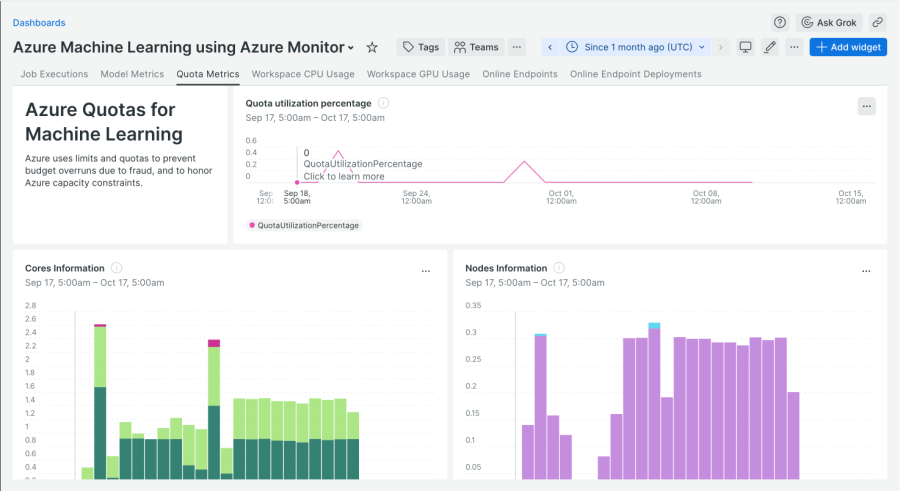
Available and used quota information for a model
The two screenshots above show how comprehensive the new integration’s dashboard is, which includes a number of tabs that provide detailed views of model metrics, CPU and GPU usage per workspace, and endpoint-specific resource utilization metrics.
In addition to the new dashboard, workspaces and endpoints are captured in the entity explorer view, providing information about deployment details, endpoint-specific traffic, and latency. The integration also offers logs monitoring of Azure Machine Learning models in New Relic, capturing such details as container name, container image name, and information about Azure Machine Learning resources.
Próximos pasos
To monitor Azure Machine Learning with New Relic, visit our docs, deploy the quickstart dashboard, or sign up for a free account (100 GB/month of free data ingest, one free full-access user, and unlimited free basic users).
Las opiniones expresadas en este blog son las del autor y no reflejan necesariamente las opiniones de New Relic. Todas las soluciones ofrecidas por el autor son específicas del entorno y no forman parte de las soluciones comerciales o el soporte ofrecido por New Relic. Únase a nosotros exclusivamente en Explorers Hub ( discus.newrelic.com ) para preguntas y asistencia relacionada con esta publicación de blog. Este blog puede contener enlaces a contenido de sitios de terceros. Al proporcionar dichos enlaces, New Relic no adopta, garantiza, aprueba ni respalda la información, las vistas o los productos disponibles en dichos sitios.